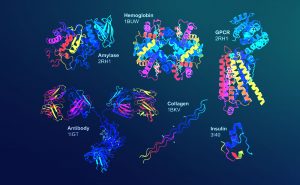
Blog
Proteome enlightenment: AI annotation for proteins with unknown function
The gulf between known and unknown proteins Proteins perform a numerous functions in living organisms.
The gulf between known and unknown proteins Proteins perform a numerous functions in living organisms.
This blog post will be focused on AlphaFold3 (Abramson et al. 2024) use for biologists.
Scientists and researchers in computational biology, particularly those involved in protein design, use tools for
How structural similarity points the way in drug design Computational structural biology can't exist without
The latest version of DiffDock, DiffDock-L (Corso et al. 2024), improves accuracy by up to
good, bad, and ugly DiffDock for drug discovery: good, bad, and ugly Molecular docking plays